At Seedcamp we first invested in machine learning as part of Fund II in 2012, when artificial intelligence was mostly confined to academia. Our earliest investments were James Finance and Elliptic, which recently raised a Series C to continue powering financial crime detection. AI fundraising activity gathered momentum in the lifetime of Fund III. We are deploying Fund VI, which we launched in May this year,
We are now deploying Fund VI, which we launched in May this year, and are particularly excited about startups building AI-driven solutions across industries and verticals. Our most recent commitments include the AI governance platform EnzAI and AI-powered automation software AskUI.
Accelerated by rapid innovations in Generative AI, the AI space has reached blistering speeds and represents 18% of all investment in the venture industry.
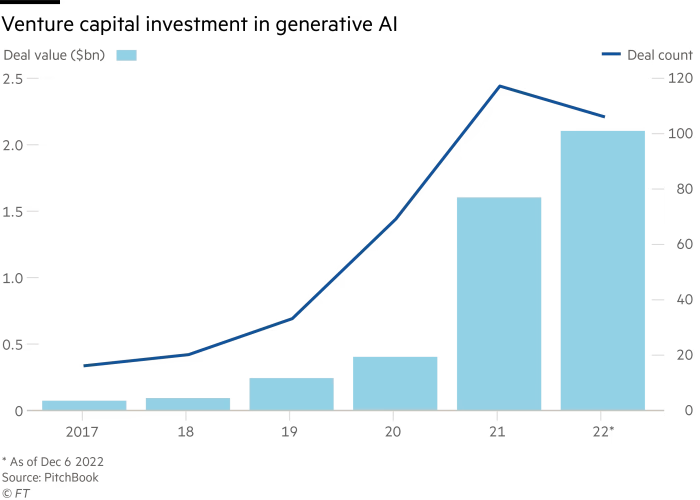
This article walks through some of the micro-themes that bind the 70 commitments Seedcamp has made to date in AI and ML.
- Applications that ‘do work’
- Enterprise workflow stack and automation
- MLOps
- DevOps and deployment
- Guardrails
- Sector-specific discovery engines
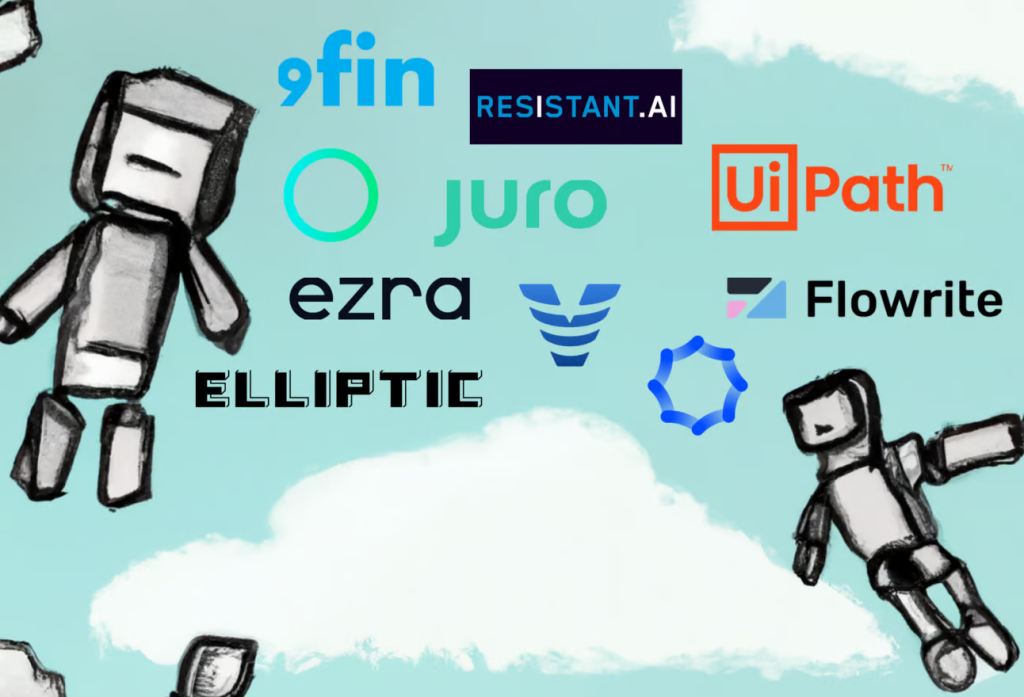
Applications that ‘do work’
Large language model are the first AI tools capable of doing work that would otherwise have been done by humans. Sarah Tavel of Benchmark has written a great article about this point of inflection. Where software could only previously enhance the efficiency of work, it can now literally do or generate it.
Seedcamp has invested in Metaview which writes interview notes, Flowrite which can write emails and messages across Google Chrome, and Synthesia which automates video production and recently achieved unicorn status.
Users can enter text to Synthesia, choose an avatar trained on actors, and generate videos
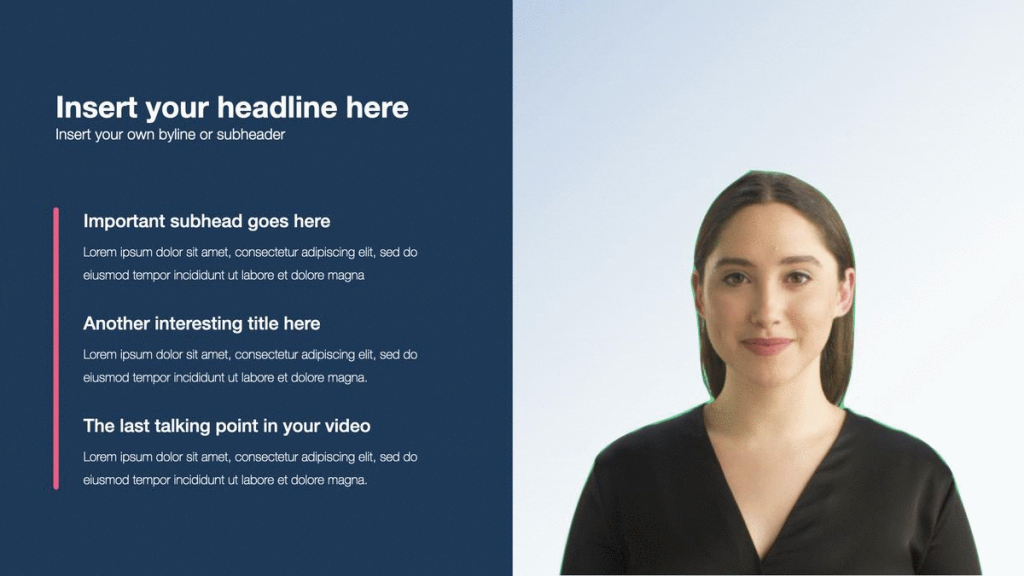
Users can enter text to Synthesia, choose an avatar trained on actors, and generate videos
The concept of software that does work is already catalysing a wave of startups and new business models and philosophies for the enterprise.
How should we re-calibrate ‘productivity’, if it is a function of infinitely re-produceable software?
Where ‘time’ was the denominator of the traditional productivity equation, [productivity = rate of output/rate of input], is the correct denominator now GPU?
If in the future humans are just responsible for validating model accuracy and precision, then the real opportunity is to find the ‘fast moving water’ (as coined by NfX), where AI is capable of the biggest volume of work.
Within this early wave of LLM applications that do work, a pattern is emerging of companies focused on automating time-consuming, high-complexity activities that are conducted in written natural language. Procurement RFPs, compliance questionnaires, legal documents, and code generation are all obvious early use cases. Companies that can translate the description of a problem in these areas to sound and reliable output are likely to capture value.
Enterprise workflow stack and automation
The lion’s share of enterprise AI applications in the last decade have focused on employee efficiency. The most remarkable innovations have been horizontal software that tackle ‘hard and boring’ problems such as process mining, application automation, and project management to give employees more time to do more work.

UiPath (NYSE: PATH) is a category leader in the enterprise work stack after going public in 2021. The company helps businesses automate and improve high volume and low complexity tasks within the enterprise and recently acquired communications automation platform, re:infer, also Seedcamp-backed. We have also invested in AI businesses automating other corners of the enterprise such as Rossum, a document gateway for business communication, Juro, a contract collaboration platform, and tl;dv, which records meetings and helps you tag important moments on the fly.
Newer robotic process automation products and features increasingly include ‘self-compounding’ elements that use previous activities to prioritise future activities based on ROI and other key metrics.
In the longer-run, there may be a significant difference between apps that ‘do work’ and more general efficiency-enhancing enterprise software. AI businesses in the former category may use models trained on sector specific data, which are necessarily vertical software (a co-pilot for every industry). In the latter category, the market opportunity includes all business with processes, for which broader approaches may be more relevant. Vertical or sector-specific winners can of course still be massive businesses, and may capture 60%+ market share whereas horizontal winners rarely get more than 15%.
MLOps
‘MLOps’ has become a nebulous branch of the AI taxonomy but remains the best way to describe companies that enable the running, maintaining, analysing and fine-tuning of AI models.
MLOps has several layers that are each prerequisites for model development; the GPU layer, the data layer, the observability layer, etc. Companies typically have an insertion point that tackles one of these and move on to add features that target several at once where there are obvious adjacencies. MLOps companies are the ‘picks and shovels’ of artificial intelligence.

Pachyderm’s products serve customers across several uses case within model production
In our portfolio, Embedd.it parses data from ML datasheets to generate drivers for semiconductors and Fluidstack aggregates spare capacity in datacenters to rent out to customers.
The speed of developer adoption of MLOps tools highlights that demand may be outstripping supply in this category. For example, at the data layer, there is still plenty of white space for AI-native tooling for observability, integration and transformation, and the race to build tools to debug and increase performance of models is red hot.
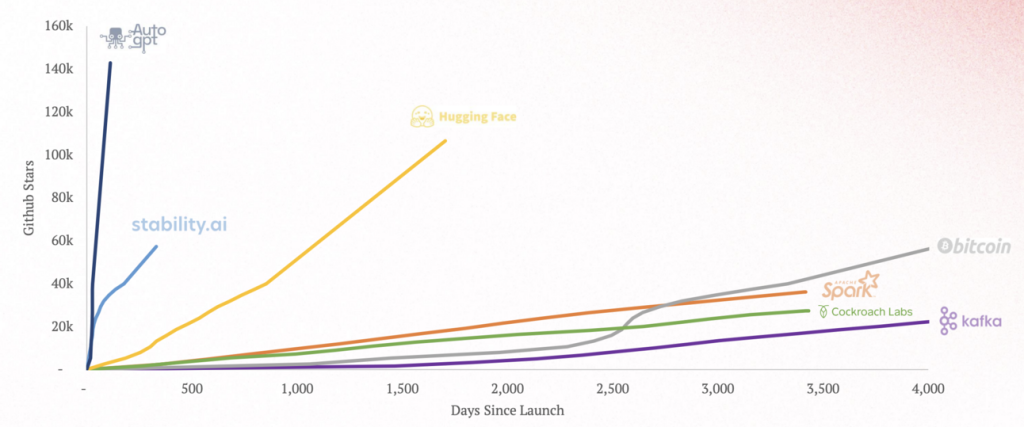
Redpoint Infrared Report (2023)
Guardrails
Propping up the MLOps ecosystem is a cluster of software trying to prevent the general misuse of AI. If generative AI is an open-road race, the guardrails are the police, the red lines, the car bumpers, the rolling barrier and the orange cones. The guardrails entail a broad set of companies spanning from cybersecurity – Resistant.ai protects AI-native systems from complex fraud and sophisticated machine learning attacks through to governance; Enz.ai helps organisations adopt policies to build and deploy AI and track their practices against them.
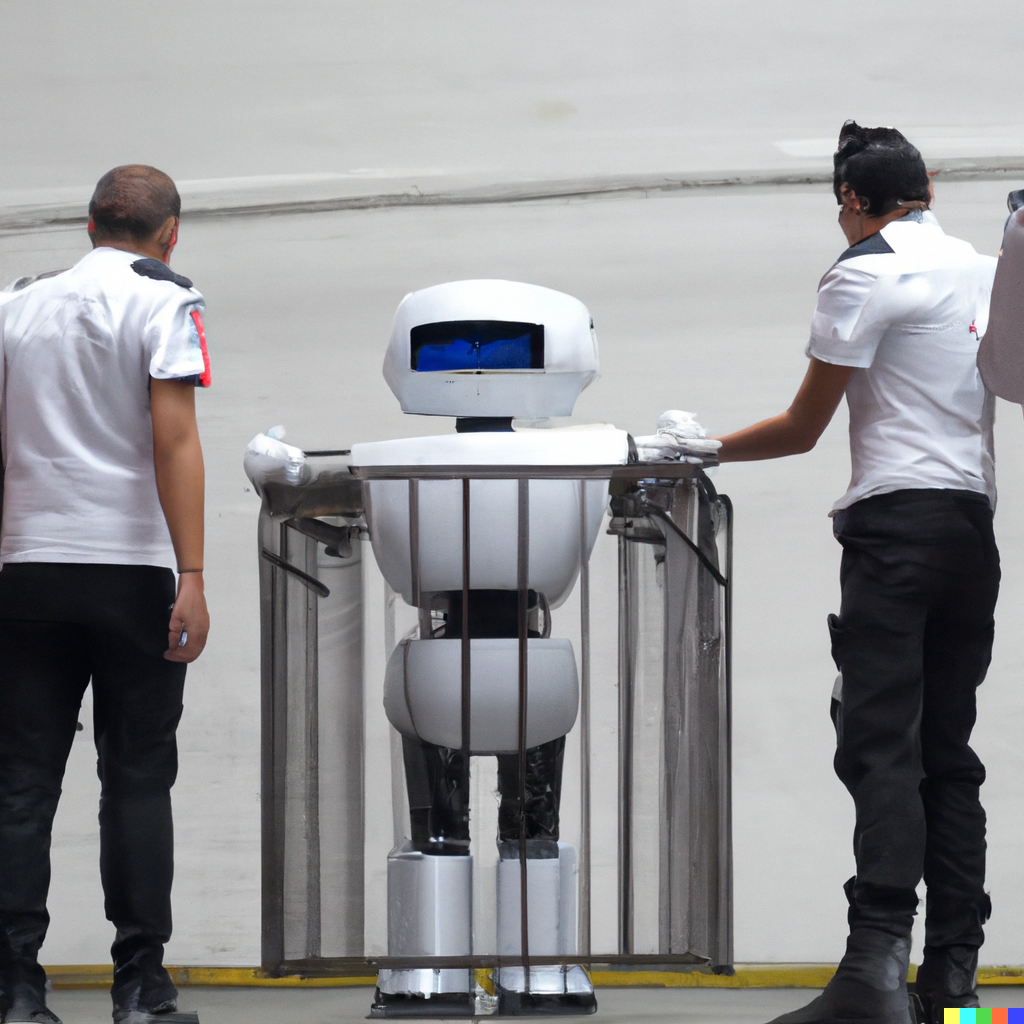
DALLE’s best impression of AI guardrails
Guardrails are plausible at many insertion points within a model pipeline. Software that ensures only the right company data is sucked into in an open-source model, software that sits on top of a model to ensure brand alignment and software that generally ‘troubleshoots’ the software supply chain in complex cloud environments, such as RunWhen.
Tools of this nature are increasingly integrated into infrastructure rather than at the application level. They have ‘shifted left’ chronologically. This enables companies to ensure compliance and security across the entire model production sequence. Although this approach often has a higher rate of false-positives, since checks occur prior to project completion, there is scope for reinforcement learning layers to drive a smoother workflow and categorise issues as they are flagged.
Devops and deployment
The hardest category to succinctly compartmentalise is the tooling layer that helps developers integrate models. These aren’t a feature of model ‘building’ but rather how developers within enterprises can best select and use these models.
These tools are often modular and focus on how models interface with the enterprise and with the end-user. Deployment channels will be especially relevant for industries with fewer software developers to conduct bespoke model integrations. Companies in the Russell 3000 might well be thinking about how to do this effectively.
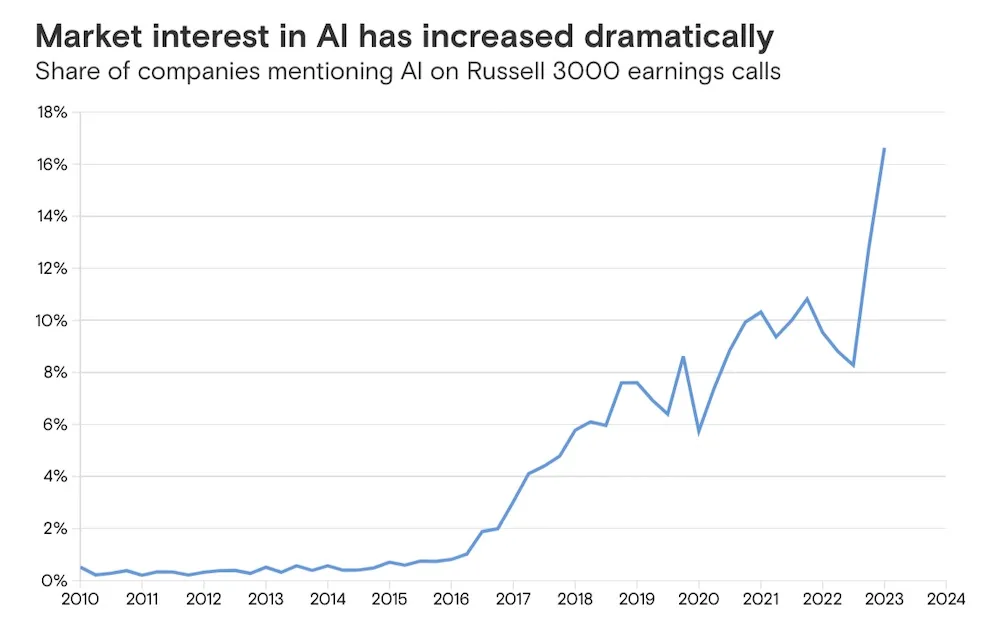
Goldman Sachs (2023)
This is one of the spaces that is moving incredibly quickly in the current LLM wave as the answers to many questions are yet to emerge.
- Will all businesses need to integrate an LLM?
- Do all sectors require a co-pilot based on industry-specific data?
- Do all employees need to be able to interface with a model or only developers?
At Seedcamp, we have invested in several early-stage businesses in this space. Dust is a platform for creating sophisticated processes based on large language models and semantic search. AskUi is a prompt-to-automation platform that understands applications and helps users build UI workflows that run on every platform. Kern.ai helps companies connect external models to a internal data in a secure fashion.
Sector-specific discovery engine
Finally, there are the predictive applications of ‘old-school’ AI; much less a la mode. This type of business typically detects unknown unknowns, such as suspicious trading patterns, but can also detect known unknowns earlier in a workflow, which is often the case in a medical setting.
Despite the heady excitement around generative AI, predictive AI still has a lot of room to improve its own value proposition. For example, intelligent AML, KYC, and transaction monitoring technologies are still trying to crack financial crime and scams. The amount of risky and illicit crypto flowing into financial platforms is still in the hundreds of billions despite trending downwards YoY and represents 2-3% of all inflows.
At Seedcamp, we have focused on healthcare and fintech in this space to-date. In healthcare, we are investors in Ezra, an early cancer detection technology that combines advanced medical imaging technology with AI, and viz.ai, which alerts care teams to coordinate care, connect professionals to specialists and facilitate communication. In fintech, we are investors in 9fin, a platform for financial institutions to leverage analytics and intelligence and Elliptic.
Other interesting areas in this space might include agriculture, manufacturing and logistics. There has been compelling innovation but it has largely been confined to incumbents like Siemens and John Deere. John Deere spent $1.9bn on R&D in 2022, or 4% of revenue. That wouldn’t be much for a pharmaceutical company but its a hell of a lot for a heavy machinery business.
One reason that AI is so exciting is that it continuously forces investors to reconsider what they believe. Seedcamp’s first AI/ML commitment in 2012, James Finance, built fintech infrastructure to determine customer eligibility. In Europe, Lendable and Funding Circle became breakout companies in this space, but AI advancements didn’t propel a large group of winners in the intervening period and investor theses about the space decayed.
With each evolution of AI technology, the category has changed and in 2021 and 2022, predictive fintechs like Marshmallow reached significant scale with technology that determines customer eligibility. Investor sentiment pivoted again✝.
At Seedcamp, we are optimistic about the way AI upends sectors and we are always interested in new solutions at every interval of the software value chain.
We are especially excited about entrepreneurs with a differentiated perspective on the future. That might be that every company will operate myriad small open-source models via a routing layer, that the entire data pipeline needs to be rebuilt or that federated learning between farms will unlock a new wave of agricultural prosperity. It might even be that language models are a flash in the pan until they are in the hands of humanoid robot butlers, doctors, and waiters.
Whatever it is, we would love to hear it, so please get in touch!
✝Funding Circle, Lendable, and Marshmallow are not Seedcamp companies.